CAPABILITY
People Analytics
Unlock the potential of your workforce with our People Analytics services. By harnessing data-driven insights, we help you make informed decisions that enhance employee performance, improve retention, and drive your business success.
CONTACT USfeatures
Our main advantages
Improved Decision Making
Make better, data-informed decisions regarding hiring, development, and retention strategies
Enhanced Employee Engagement:
Understand what motivates your employees, what doesn’t, and how to boost engagement levels
Reduced Attrition
Pinpoint the factors that lead to employee attrition and develop strategies to retain top talent.
DELIVERABLE
Advanced Data Collection
Gather comprehensive data from multiple sources within your organization for a holistic view of your workforce
CONTACT US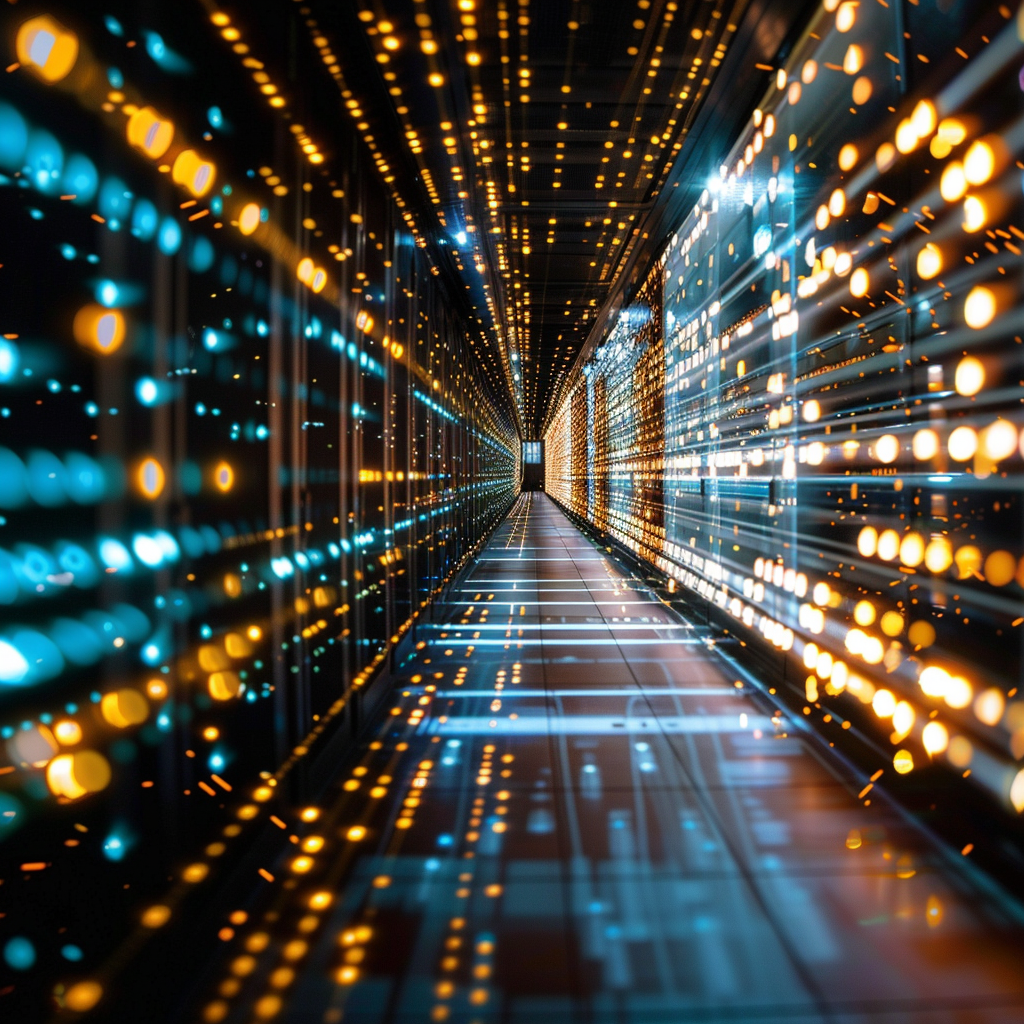
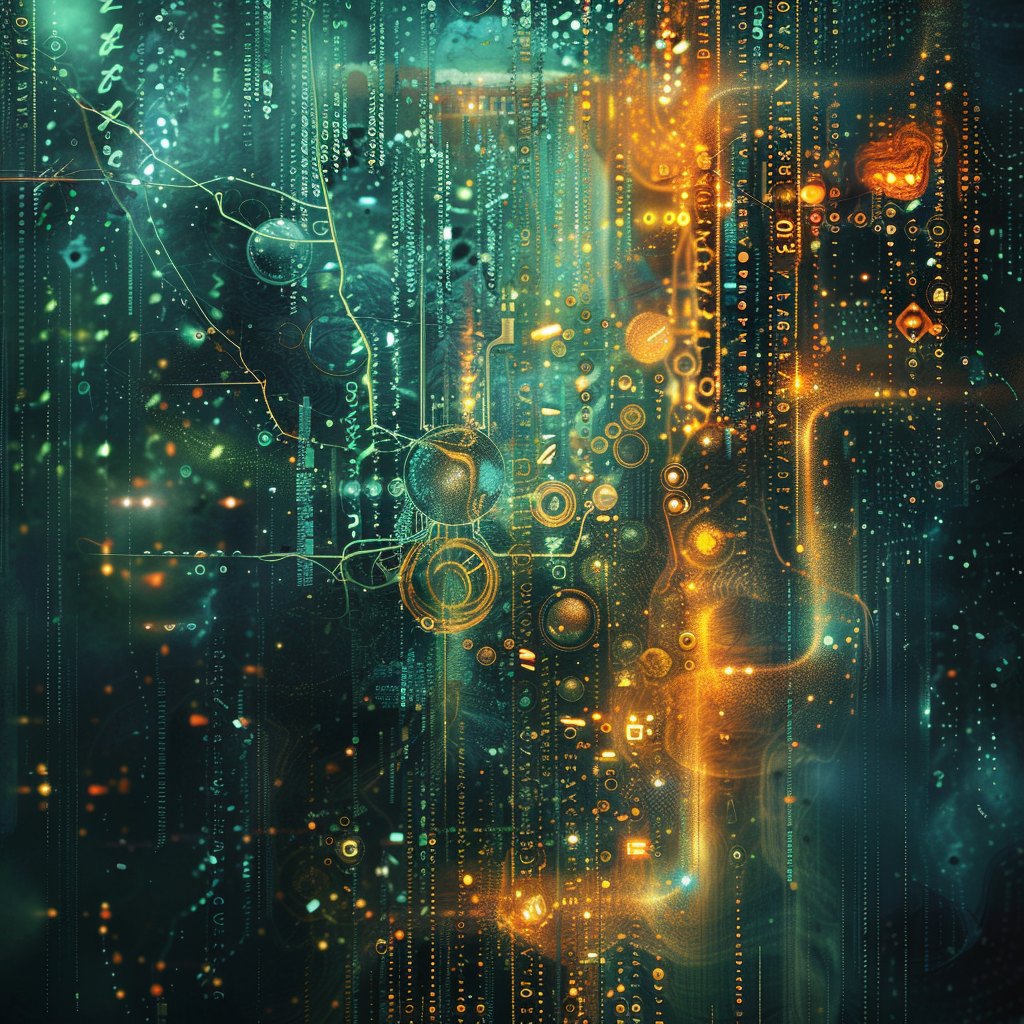
Connect with us today for inquiries on how we can help.
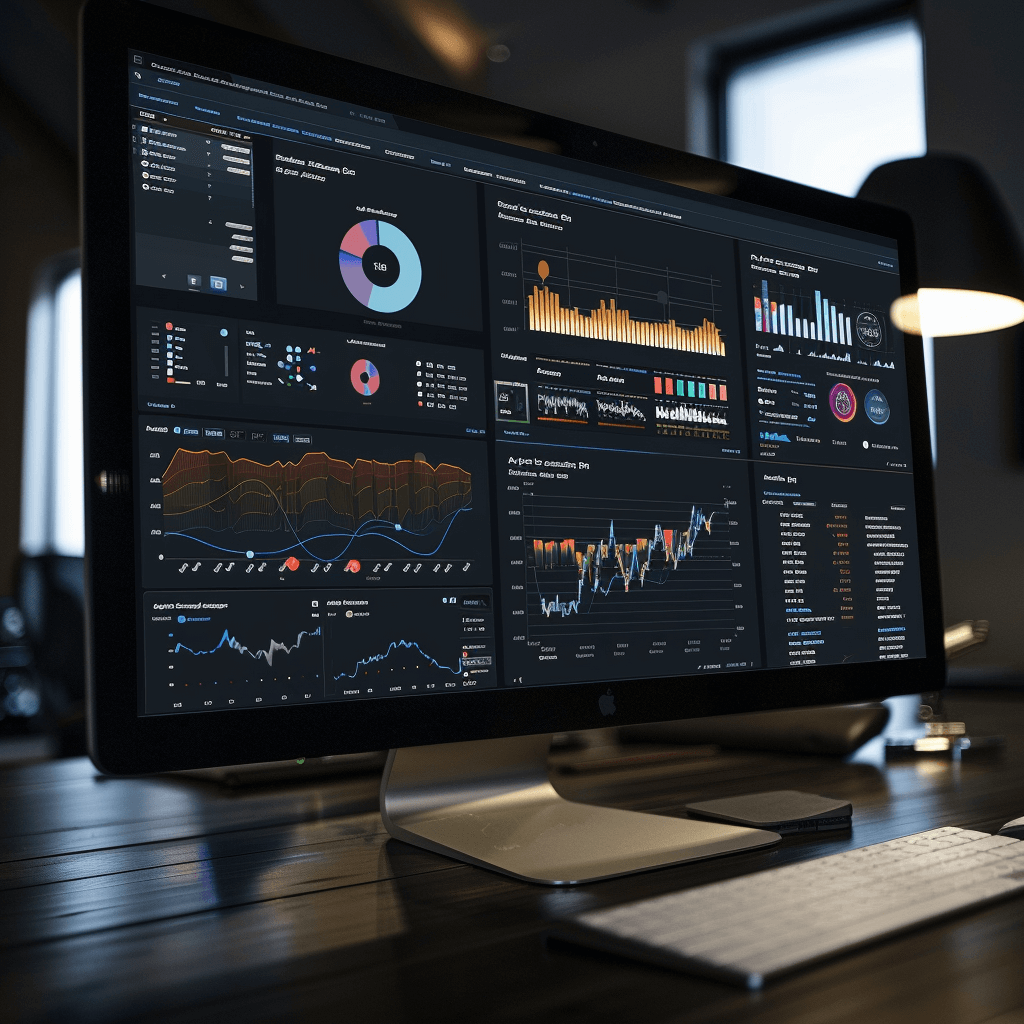
DELIVERABLE
Analytics Implementation
Deploy advanced analytical tools to sift through data and identify patterns and trends.